If artificial intelligence (AI) is properly deployed, it improves credit unions’ fraud decisioning capabilities, Provenir’s EVP for North America Kathy Stares believes.
Fraud detection and prevention is a top priority for credit unions as they consider how AI can improve service delivery, likely because fraud hits them hard. According to a recent survey, 79% of credit unions and community banks reported more than $500,000 in direct fraud losses, more than any other segment. According to Juniper Research, businesses across the globe will spend more than $10 billion every year on AI-enabled financial fraud detection and prevention strategy platforms in 2027. That is a more than 50% increase from 2022.
AI is an optimization tool
Stares said predictive AI enables financial organizations to optimize business processes. That frees up resources and fosters a more focused approach to fraud. AI can process millions of attributes beyond human capability to deliver predictive capability effective in fraud modelling across the customer life cycle.
“There are trends that are identified, and the ability to operationalize those in a decisioning platform, I think, is what’s key,” Stares said.
Credit unions have unique AI considerations
By design, credit unions can attract different fraud types. Their branch and membership design lends itself to first-party and identity fraud. That also attracts social engineering scams.
As they integrate digital fraud prevention solutions, credit unions must maintain high trust with their localized base. Systems must decrease false positives and allow legitimate clients to transact seamlessly. Stares said AI-based systems must be coupled with real-time decisioning to deliver early identification and warnings.
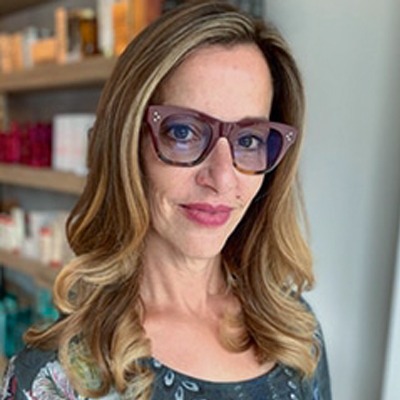
Credit unions tend to have more homogenous client bases. AI is perfect for quickly identifying aberrant behavior. As the model is fed more data, it will identify suspect activity much more rapidly.
“It’s important to be coupled with alternative data,” Stares advised. “That’s coming in and matching to see if it has any predictive nature in identifying fraud across the lifecycle like bust out fraud. Putting in KYC and AML data, potentially using transaction-based data where customers allow you to look at their actual bank accounts and financials, will be indicative of things that can move future fraud.
“That’s why the technology is important to inject data in a real-time fashion so that you can use the data… to enhance models or potentially put models in that you can champion/challenger to look at the predictive nature of preventing fraud across the lifecycle. So early warning is key.”
Fraudsters also use AI. It helps them quickly pivot to new strategies as institutions catch on to their tactics. Credit unions can use it for the same purposes: to quickly identify suspect activity before it’s written off as a collection.
Scaling considerations
AI also plays a role as credit unions consolidate and gain scale. Stares said it is essential for AI to be connected to all relevant databases, considering false positives and looking at everything in totality. Data is key. Develop proficiency in injecting data and then using AI to quickly detect fraud.
“I don’t think size matters,” Stares said. “Size and scale may bring different types of fraud attacks and in number of fraud attacks, but I think the way you handle them is the same with using AI with alternative data injection and real-time processing of models.
“If you have the right technology, and you’re able to connect to all the silos and put in other data, run them through your decision strategies the same and treat them in the same manner because you you have consolidated the data. I don’t think there is a significant risk. If you’re unable to do that, there may be risks in treating each population because you could have to treat each population differently. And you may have a risk of higher false positives.”
Predictive AI and the human touch: Important considerations
While Generative AI is generating more buzz, institutions are wise to first consider predictive AI. Stares said it can help test the effectiveness of different fraud detection models. Which ones create more false positives, for example? AI-based models also learn from their mistakes and improve over time.
And while the human touch has its place, Stares said it can also hamper AI’s effectiveness. Human intervention is based on experience. If models become too backward-looking, their predictive power is diluted.
Optimal customer experience must also be maintained. Loyalty ain’t what it used to be.
“Loyalty to a financial institution is not what it once was,” Stares observed. “But if you can provide the experience and provide all of the points of product in one place, your consumer is likely to stay there.
“So you can’t transfer the risk onto the customer experience. You need to use the AI and the data to mitigate fraud or credit risk without impacting the customer.”