Enjoying our podcasts? Don’t miss out on future episodes! Please hit that subscribe button on Apple, Spotify, YouTube, or your favorite podcast platform to stay updated with our latest content. Thank you for your support!
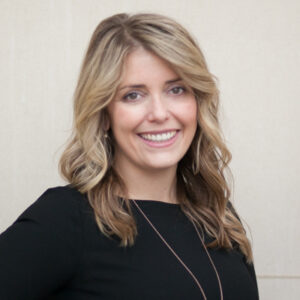
The use of machine learning models in underwriting for consumer loans has been around for more than a decade. While fintech clearly took the lead here it has really only been the last couple of years where traditional lenders are engaging with this technology. While these AI/ML models are different from the Generative AI craze that has embraced the business world in the past year, this phenomenon has certainly helped with awareness.
My next guest on the Fintech One-on-One podcast is Laura Kornhauser, the CEO and Co-Founder of Stratyfy. Her company is on a mission to enable greater financial inclusion for people while also helping financial institutions better manage and mitigate risk. They do that by implementing their advanced AI models and, of course, through the dedication of their people.
In this podcast you will learn:
- The founding story of Stratyfy.
- The company’s mission and how it has evolved?
- The different types of financial institutions they work with today.
- How they differentiate themselves from others in the space.
- How their UnBias product works.
- What being transparent means for adverse action notices.
- What is top of mind for most banks and fintechs today.
- The types of data their clients are using that are most important.
- What is involved in implementing Stratyfy into a lender’s system.
- How their AI models have improved over time.
- How the popularity of AI through ChatGPT has impacted Stratyfy.
- What it was like raising an equity round in 2023.
- The biggest challenge Stratyfy is facing today.
- How AI will continue to improve when it comes to credit and risk decisions.
Read a transcript of our conversation below.
Peter Renton 00:01
Welcome to the Fintech One-on-One podcast. This is Peter Renton, Chairman and Co-founder of Fintech Nexus. I’ve been doing this show since 2013, which makes this the longest running one on one interview show in all of fintech. Thank you for joining me on this journey. If you liked this podcast, you should check out our sister shows The Fintech Blueprint with Lex Sokolin and Fintech Coffee Break with Isabelle Castro, or listen to everything we produce, by subscribing to the Fintech Nexus podcast channel.
Peter Renton 00:39
Before we get started, I want to remind you that Fintech Nexus is now a digital media company. We have sold our events business and are 100% focused on being the leading digital media company for fintech. What does this mean for you, you can now engage with one of the largest fintech communities, over 200,000 people through a variety of digital products, webinars, in-depth white papers, podcasts, email blasts, advertising, and much more. We can create a custom program designed just for you. If you want to reach a senior fintech audience, then please contact sales at fintech nexus.com today.
Peter Renton 01:21
Today on the show, I’m delighted to welcome Laura Kornhauser. She is the CEO and co-founder of Stratyfy. Now Stratyfy is a super interesting company that focused on AI based risk decisions for lenders, we actually obviously talk about what that all means. We spend also a lot of time talking about bias and how stratifies models really help identify bias. We talk about transparency, and how that is built into everything that they do as Stratyfy. We talk about the different types of data, we’ve talked about how their models have improved, what’s involved in implementing Stratyfy into a new lender. We also talk about just AI in general and why it’s it’s been such a hot topic and how that’s impacted them. We talk about funding rounds, and much more. It was a fascinating discussion. Hope you enjoy the show.
Peter Renton 02:20
Welcome to the podcast. Laura.
Laura Kornhauser 02:22
Thank you so much, Peter. Happy to be here.
Peter Renton 02:23
All right. Great to have you. So let’s kick it off by giving the listeners a little bit of background about yourself. I know you had a decent stint at JPMorgan Chase, it seems like tell us some of the highlights of your career to date before Stratyfy.
Laura Kornhauser 02:39
Wonderful. So yes, I started my career at JPMorgan Chase, I spent over a decade there in both lending and risk roles in the institution, which is where I uncovered many of the problems or solved firsthand many of the problems that we address here at Stratyfy. Prior to that I am an engineering undergrad. I studied machine learning in my undergrad degree before it was called that. It was just called advanced statistics back then. And then, you know, when I was transitioning out of JPMorgan, when I decided to leave, I very much had the hopes and dreams of starting a company. You know, my parents are entrepreneurs. They started a business around the time I was born and then built and grew it into a multinational business, and they eventually sold to a strategic. So those were my true I guess, first jobs, starting from you know, answering the phones when I was in high school all the way up to network editing when I was in college. So, you know, I always had that entrepreneurial, if you will, spirit within me. Went the completely other direction, as many would say third children often do, out of undergrad, but then very much knew I wanted to return to that home and be a founder.
Peter Renton 03:49
Okay, so let’s talk about the founding story, then of Stratyfy. What specifically did you see and what are you trying to solve?
Laura Kornhauser 03:58
Absolutely. So interestingly, after leaving JPMorgan, I had an experience, a personal experience where a credit card product was heavily marketed to me actually by Chase of all people, and it had a great points plan and I’m a sucker for a good points plan. And I signed up for the credit card and I was rejected. And that then led me to, you know, call the number on the back of my rejection notice, talk to someone that that I provided some additional information to, and then literally, you can almost hear the boop boop, boop, boo, boo boo boo in the background, and I was actually approved over the phone. And that experience for me really opened my eyes to the way in which credit decisions are made by so many institutions, and the large groups of people that are left out from those decisions. You know, I was in a fortunate place. I didn’t need that credit card. You know, it was not something that was going to materially change my life. But for many other folks tHow hese types of credit products are, you know, help them buy their first home, help them, you know, fund inventory for their small business, you know and have really meaningful impact and, and that that was something I really wanted to address, I was fortunate around the same time to meet my co-founder, Dmitry Lesnik. And he had been spending the prior decade before us meeting, developing a family of algorithms that’s still at the core of the technology and services we provide at Stratyfy. And what’s really nice about that family of algorithms is it enables you to learn from data automatically scalably, but in a way that is highly, highly transparent to the user. So I saw the application within credit, and within other highly regulated use cases where you know, I in my previous life at JPMorgan had even struggled to get the right technology to to fit the problems that we were trying to solve.
Peter Renton 05:54
Okay, so then, fast forward to today, you founded in, was it 2017? Six years ago, now, tell us a little bit of how the company has evolved and how you how you describe the company today?
Laura Kornhauser 06:05
Yeah. So when describing the company, I start with our mission, which is has been our mission since since the get go, which is to enable greater financial inclusion for people while also helping financial institutions better manage and mitigate risks. We see it as two sides to the same coin, we can’t do the first without doing the second, or we can’t do the first scalably without also doing the second. So when we started the company, we were very focused on credit risk scoring and credit risk decisioning. So helping lenders understand the true risk of borrowers, primarily consumer and small business borrowers, helping them understand that true risk and make more informed decisions based on on those enhanced risk predictions. That yes, leveraged insights from data in an automatic way, but did so in a way that still allowed a non data science user to understand what the heck was going on, which we see continued to see is really important. Fast forward to today, there has been a ton of focus in the industry, not just on AI and machine learning over the last in particular year or so. But a tremendous focus on the industry about how technology can be leveraged, but in a safe and sound and fair way. And we are perfectly positioned for that. I would argue that maybe when we started the company, we were still a little bit early for the market. But the growth trajectory that we’ve seen, particularly over the last 18 months has really been unbelievable, and also allowed us to expand into other use cases. So right now, we also have customers in fraud detection, where we’re helping them identify fraud, ensuring fairness, and reducing false positives along the way. And then we also pulled out our bias detection and mitigation capabilities into a separate solution that we call UnBias. that focuses square on, squarely on fair lending risk assessment, and enables lenders to do that more efficiently, more proactively and identify risks before they become problems.
Peter Renton 06:07
I wanted to get a sense of who you’re working with. What are what are some of the financial institutions, what types of financial institutions do you work with right now?
Laura Kornhauser 08:13
Yeah. So we started off working primarily with fintechs. So those were early adopters, our initial customers and enabled us to get some really unbelievable product feedback and quick iteration cycles on our offerings. Now, we’re working with banks. And we’re working with banks, actually, from a pretty wide spectrum right now, our largest banking customer is a top ten bank in the US. And then we’re also working with smaller community banks and a number of CDFIs. Most notably, through a recent initiative we launched, called underwriting for racial justice and the pilot program that we’re the technology partner for that we can definitely talk more about. But we see a huge opportunity in the CDFI space, in particular, we see a huge opportunity in community banks, for technology like ours, and then we’re also seeing quite a demand from the you know, I would say big community banks transitioning into regional banks as well.
Peter Renton 09:11
Okay, so then you’re not in this space alone. There are others that are also providing services to those kinds of financial institutions. How are you different from others in the space?
Laura Kornhauser 09:24
Yeah, so where we really differentiate ourselves is in the level of transparency that we provide into both models or scoring systems and decisioning systems. So that has become a little bit of an overused buzzword where everybody claims to have transparency. You know, when we say that we mean that our users have full visibility into the inner workings of how a model or strategy works. They also have the power to make changes and do so you know, without writing a single line of code, we find that that ends up being really meaningful, especially for, again, the community banks out there, and even many of the regional players that, you know, if they have a data science team, it may be a few people if that they’re really stretched, overworked. And what we really are focused on doing is how do we bring the tools of data science, to the subject matter expert, to the user that really understands credit and sure is very highly competent in data and knows data but is not a data scientist is not a engineer, how do we give them tools that they can really feel comfortable using, because of the level of visibility and control that we provide versus others? So no black boxes whatsoever with Stratyfy and all that is enabled by that core technology that I mentioned earlier.
Peter Renton 10:51
Interesting. I want to touch on bias. You mentioned it a couple of times already here. It sounds like this was a really founding principle for you guys, what is your approach? Maybe you can explain exactly, you know, how your models are able to identify bias better than others.
Laura Kornhauser 11:10
Yeah. So this is something, you’re absolutely right, part of our founding approach, you know, our initial solution that we built, our credit risk assessment and decisioning solution always included bias as a KPI of models. So we always thought that that was one of the performance indicators that you should be looking at when evaluating different strategies, different options, different models. And, you know, one, what we do is, we are not in the business of saying or determining what is fair or what is not fair. What we are in the business of doing is offering a number of different tests, metrics, all of which can be easily leveraged within our tools to evaluate the potential bias that could creep into addition. So one thing we do Peters, we support a number of different bias metrics and let and let our user make the decision about what metrics matter most to them. What what metrics matter most to the regulators, their customers, and they can select those. And then the way our UnBias product works is the first step, we actually break it into three steps, uncover, understand, undo. So the first step uncover is all about running those tests, running them in a in a very robust, yet automated fashion, such that a lender can run those tests more frequently and more proactively. If a risk emerges according to one of those indicators, we move to step two, or allow the user to move to step two within our products, which is understand. There, we decompose that risk. So what are the primary drivers? What are causing that bias risk to emerge? And then after illuminating that, we are giving, you know, our customer the information, they need to determine if they need to take action. And if they decide they want to take action, we also with the undo component can help them figure out the way to remediate, make changes to their models. And correct for, or compensate for the bias that has emerged because nobody sets out to build a biased model or a biased decisioning strategy, right? There’s not a lender out there that says, hey, you know, either my humans making decisions nor my automated system, or some combination of both, as is the case that many lenders, right, nobody intends to have that bias. But we find that a lot of the robust checking that happens, happens kind of on launch, before a new strategy is launched. In the end, yes, there are periodic check ins as well. But oftentimes, things can kind of get off the rails faster than the next periodic check that comes in place. So you know, our goal with this product offering and what we’ve been able to deliver to customers is better visibility into an ongoing monitoring of those risks such that you can address an issue before it becomes a big problem.
Peter Renton 13:58
So you might see, like someone’s running your models, and there’s been say, several weeks go by and they can start to see, there’s a there seems to be like, whether it’s women, whether it’s racial, you can say right, well seems to me that you’re you’re declining more of these types of people than you should be. And so is this something that just, is there a trigger point? Or does the customer set the trigger point?
Laura Kornhauser 14:25
Customer gets to determine the frequency with which they want to run the evaluation. You know, we can do it daily, or, you know, even multiple times a day should a customer want that we find that in most cases, we’re looking at monthly or quarterly that folks want to do these checks. It’s very hard to measure. If you don’t have a sample set that is a big enough size, you can run into situations where you may flag something that is not statistically significant. So we’re really focused on you know, not just the measurement, but in ensuring that that measurement is statistically significant so that we can feel comfortable quantifying something as a risk, and we’re not, you know, throwing up a bunch of flags where they were, they don’t need to be.
Peter Renton 15:05
Right. I imagine that could be a challenge for some of the smaller community banks, right that don’t have, that don’t have that volume?
Laura Kornhauser 15:11
Exactly. Don’t have the volume to, you know, run with any more frequency, you know, then monthly if that, and often for the smaller banks, they want to run that on a quarterly basis. But you know, our technology enables them to run with whatever frequency they want, we find the market once monthly, or quarterly.
Peter Renton 15:29
Does your system also kind of help with the adverse action letters or that someone’s been declined? And obviously, we need you people need to know why. Is that part of what you’re offering there?
Laura Kornhauser 15:42
Absolutely. And it’s also something I see as a differentiator of ours, again, pointing back to the level of transparency of our underlying approach. A lot of folks that use other machine learning approaches and then provide adverse action notices off the back are using things like Shapley values to provide those adverse action notices, or the reason codes, regulators have come out and raised flags about those types of postdoc explainers. Now they haven’t said they’re not explainable enough. I think the exact language that was the postdoc explainers may not be transparent enough for the use, for this type of use. But that’s still I would say, a hotly debated item in the industry, and many folks are leveraging those methods if they’re using more blackbox, machine learning solutions. We don’t have that problem because the underlying nature of of our models is they’re interpretable, meaning they’re visible or transparent, you know, from the building blocks up as opposed to layering a model on top of the model to understand how the models working.
Peter Renton 16:46
Right, right. And then you’ve got, like you got the CFPB have made it pretty clear that they want to, they don’t want to see any bias in, in lending models. So I mean, I imagine most, if not all lenders would be pretty aware of this today. And is the bias piece something that is top of mind for lenders today, or how do you, when you’re having conversation, is this sort of the feature that they’re most interested in? Or what, what’s it like?
Laura Kornhauser 17:14
It’s an interesting market environment, I would say top of mind for most banks is grow deposits and grow deposits and then grow deposits. That being said, it is a huge focus. Banks right now, given the environment we are in, are slashing headcount, costs, etc. And looking for ways to automate processes, looking for scalability, looking for efficiencies via technology. AI and the subfield of machine learning has a ton of value to offer to drive those kinds of scalability and efficiency gains. But we find that many in the market are still fairly timid on using machine learning for these types of high value, high risk decisions with high levels of scrutiny. And you know, that’s where we’re really able to differentiate ourselves. That’s why we’ve seen the growth that we we’ve seen, is because we can offer them the benefits of that technology without some of the drawbacks, you know, without making them feel like they have to sit and blindly trust a score or model they don’t understand, they can very easily customize everything to their particular risk tolerance, their particular customer base, they see again, see exactly what was learned from data, can change it, can override, can put additional information into the system that is outside the data to compensate for things like bias, to compensate for the things you know, things like the data is always backward looking. So that, I think, has really helped us in what is ultimately a tough environment.
Peter Renton 18:52
Let’s talk about the data itself, because I would love to kind of get a sense of the kind of data that is really becoming critical to some of the things we’ve talked about here to identifying some of this bias. And maybe data that’s less important.
Laura Kornhauser 19:09
A few things on the data side, often we meet the customer where they are. And we have data partnerships, but Stratyfy itself is not a data provider.
Peter Renton 19:18
Right.
Laura Kornhauser 19:19
So you know, we are not saying hey, add this data element to your model, and you’re going to achieve analytics bliss. We are working with the data assets that they have, or data assets they acquire through one of our data partnerships, and making the best use of that, extracting maximum value from that. We still find that the majority of lenders, especially as you, you know, move into the communities, community bank space, are still using traditional credit data. What they’re looking for is a better way to extract value out of that data to achieve greater performance, greater accuracy, but you know, without sacrificing visibility, transparency, control. There’s a lot of talk about additional data elements. And many lenders, either fintech or larger lenders are using other data elements to help especially compensate for thin or no file applicants, you know, from our work, it shows, you know, tremendous profit promise in these areas. You know, I’m a big believer in rental payment data, for example, and in particular, the ability for that data to really help on the fairness side, drive down bias and help boost up some of those thinner file applicants. We’ve all seen, and I know you’ve read the studies, you know, from FinRegLab, and others who we also partnered with FinRegLab on a very interesting study on machine learning and underwriting but cash flow based underwriting, also extraordinarily promising. And again, we see different lenders at different points in their adoption curve on those alternative data. It’s always interesting to me, though, because many lenders still, when you talk about alternative data, or data outside of a credit report, think that you’re talking about scraping someone’s social media profile. Right? And I often joke, like in our space, alternative data is not that alterna, right?
Peter Renton 21:17
Right.
Laura Kornhauser 21:18
So you know, sometimes you have to kind of talk people down as you’re starting to broach that conversation. But in every one of those discussions, as I’m sure you can imagine, especially in the market environment, key question for that lender to answer is, what is the additional uplift that that data element gives? Does it justify the cost I have to, or the friction I have to introduce to get it? And we often see folks using our products to help do that test, if you will, as well to explore the value of that additional data element. The other thing I’ll mention here, Peter, is that we have seen that you don’t need 1000s of attributes to make good decisions in credit. And that oftentimes, there is almost like a point of saturation, where Yes, perhaps you’re adding marginal incremental value, but it doesn’t necessarily justify the increased model complexity, or the cost of that data. So we are not in the, like some of the others in our space of the, we look at thousands of attributes to make a decision with any of our customers right now, you know.
Peter Renton 21:18
So then when you’re, when you sign up a new customer, a new lender, what is involved in the process of implementing Stratyfy? How long does it take? Take us through a typical journey there.
Laura Kornhauser 22:35
So initial engagements typically begin with with a pilot agreement that runs for between one and three months. In that pilot agreement we exchange data, that is the lenders data that is exchanged with us, it’s all anonymized so they don’t have to share any PII with us or anything like that, which is quite helpful. And then we have conversations about if they want to explore other data assets, again, usually in pilot, that’s not something that folks are doing. And then we work with them to build an initial set of challenger models and challenger strategies, you know. So models producing a score, strategy producing a decision, right. Work with them to produce a set of challenger models and strategies within our software that they can then evaluate. Then for ongoing execution, we’re often integrating with an LOS for ongoing execution just via API. And that’s all controlled by our products so that you can easily, with the proper controls, promote a new strategy to the one deployed for an API without having to change the integration. And then we see usually lenders will roll that in, so no lender is going to, after a pilot as we move forward into a long term engagement, you know, on day one, flip everything over to the new challenger model. So usually that gets rolled in over time, starting at a certain percentage, and then kind of rolling that in.
Peter Renton 24:01
Okay, so then how have your models, how you’ve developed your AI models, how have they improved over time?
Laura Kornhauser 24:07
So great question. And it gets me to another point of differentiation, we do not take our customers data, and then create a shared repository for all that data that is then leveraged by every other customer. So our customers data remains their data, which we see is really important to them. That said, with the way that our family of algorithms work, you could think of it as features or insights that are being extracted out of the data that is ours. And that is then used to enhance or improve, you know, creating, if you will, the network effect for our company of with every new customer we get it adds value to everybody. So that’s how we do it. We purposely though, are not creating that, you know, big data repository that everybody is rolling from?
Peter Renton 25:01
Okay, so we’re coming up on a year since ChatGPT was released and everyone started talking about AI. I mean, it’s just amazing. You look through any, any newspaper today, and there is AI, there’s AI articles every single day, everyone’s talking about it. Has that changed your approach? Has it made it easier to kind of, or more difficult to kind of explain what you’re doing?
Laura Kornhauser 25:26
Fantastic question. The answer is, it has increased the conversations around the topic, and I think created almost two camps within financial services. And you could slightly correlate these camps to the asset sizes of the institutions they work with, but it wouldn’t be perfect. And the one camp, sees the promise, sees the value, sees the risks, of which there are many, sees the risks as well, but wants to figure out, and in many cases needs to figure out, you know, a number of institutions that we work with, you know, have a indirect mandate from their board to figure out a way to leverage this technology. So they have a real desire to figure out how to make it work for them. With a healthy, I would say, healthy dose of fear. The other camp is just been too inundated, you know, sees the word AI, and immediately turns off. And, you know, as I’ve been active on the conference circuit, as many others have for the past two months, and it has been very interesting to me to see, people are not in between, or I have found very few in between, they fall in one of those two camps. You know, I believe very strongly in the power that AI technology, broadly speaking, has to bring to the finance industry. If you understand that with great power comes great responsibility. And, you know, these tools can be used to make things a lot better, especially in the issues of fairness. They could also be used to ingrain bias and scale bias exponentially into decisions going forward. And we’re at, I think, an inflection or decision point where, you know, I really hope it goes the former way. But if we don’t have the right controls in place, controls that don’t stifle innovation, but controls, we could have a situation where all the biases of the past become encoded in the decisions of the future.
Peter Renton 27:29
Right. So I want to switch gears a little bit and talk about raising money, because when we last chatted, you were just, I think you’d just closed your your funding round. And I don’t know if it was public yet, but you had just closed it. Congratulations! It’s not easy to close a funding round in 2023. So tell us a little bit about that process, who are your investors and how that process went?
Laura Kornhauser 27:51
Well, it was a very challenging fundraising environment, there’s no question about that. But we are very fortunate to have investors that both share our mission and values, but also see the tremendous upside for Stratyfy. You know, we benefited strongly from having relationships over the long term. Right, we have been around for a while. And we have been nurturing relationships with investors for a while. And that then meant that when we were going out to fundraise, we were actually, as shocking as it seems given the funding environment, doing it opportunistically. We were raising at that time, not because we were running out of money, but because we had customers that we had either signed, or were about to sign. And we needed to make sure that we could scale the team to meet the engagements that we had landed. So also being in that position, put us in a greater, of course position of strength to fundraise. But we wouldn’t have been able to do it without those long-term relationships and without investors that really care about driving a fairer financial system and believe that Stratyfy is a key component to making that happen.
Peter Renton 29:10
Okay, so then, looking at your business today, what’s your biggest challenge to try and grow Stratyfy?
Laura Kornhauser 29:17
One thing that is a challenge right now and it’s always challenging, selling into banks. Not an easy thing to do.
Peter Renton 29:25
Right.
Laura Kornhauser 29:26
Not an easy thing to do. Sales cycles are long. Contracts are lumpy. We went into this, you know, eyes open. It’s not as if this was a surprise to us. We knew that that was a challenging path that we were going down. But that’s hard right now. That’s hard right now in the market environment that we’re in right now. And a lot of lenders are cutting back on risk, closing down products, and they in many cases are doing it with very blunt instruments, raising a FICO cut off. Completely closing down a certain offering or completely selling off that offering to the secondary market, right? We see that as an initial reaction that will pass and also create tremendous opportunity, especially for community banks and regional banks that for so long had been squeezed by fintech lenders on one side and large banks in the other. So we believe it’ll create a really meaningful opportunity. But right now, that is a challenge. What I am really focused on in addressing that challenge is, you know, a classic control the controllables. We have an unbelievable customer base today, continuing to deliver to them in the highest quality way possible, will give us new opportunities to expand with that existing customer base. And then I’m really focused on our team, we have built an absolutely unbelievable team. I’m very proud of the fact that that is, you know, a female lead team as well, which is a massive differentiator, if you will, in the market environment that we’re in, I’m very proud of that. But you know, beyond any one thing that could classify any of our employees, I’m really proud of how committed they are to our mission, how passionate they are about the change that we’re looking to drive, and how hard they are working to deliver on that. So, you know, I’m really focused on growing that amazing team that we have to continue to meet the new market demand that that we we will eventually face and weathering whatever challenges we have on selling into banks in the short term.
Peter Renton 31:30
Okay, so let’s end with with a forward looking question and want to kind of get your sense of where we are today. I mean AI continues to improve, How is this going to develop when it comes to credit and risk decisions for lenders? What does that look like in five years time?
Laura Kornhauser 31:49
Yeah. So I believe very strongly, that we will have a lot more automated decision making in lending. It’s not to say that certain decisions won’t still require manual review or won’t still require a second set of eyes, but automated decisioning needs to proliferate further than it already has. And that’s going to happen across different product lines. But what I think is really important, and this goes to the future of AI and credit and other places, is that the types of systems that are going to win, that are going to provide the most value to customers are systems that allow for input from ultimately multiple sources. So that could be data as one source, but also humans, who…Machine learning is really good at eating data and finding insight. Humans are really great at applying context to that data, information that is outside of the data elements. So I believe if you will, the AI of the future, especially for regulated use cases, but I think it for other use cases as well as the public awareness of AI system grows as we get new regulation likely coming over and kind of following a lot of the regulation that we’ve seen in Europe, and we’ve already seen the initial stride with that with 1033, there’s going to be a real focus on how do I understand what is happening, not just from data, but also from people? Combine those two into one automated system, and ensure that I can tell the FI, or the other type of business can tell their customer on the other side, what the heck happened? How was this decision made? What information was used? How can I help you get to a different decision, which I continue to believe is a huge opportunity for a case where you have a negative outcome? How do you build a relationship with that customer to help them get to a positive outcome? You know, it’s going to be it’s going to be AI systems that can do that, that are going to actually deliver on all of the promise and all of the value that we hear about in all the newspapers.
Peter Renton 33:47
Okay, then we’ll have to leave it there. Laura, thank you so much for coming on the show today. Best of luck to you.
Laura Kornhauser 33:53
Thank you so much, Peter.
Peter Renton 33:57
Well, I hope you enjoyed the show. Thank you so much for listening. Please go ahead and give the show a review on the podcast platform of your choice and go tell your friends and colleagues about it. Anyway, on that note, I will sign off I very much appreciate you listening, and I’ll catch you next time. Bye.