The importance of liveness detection was one positive development arising from the COVID-19 pandemic. It’s an essential feature of a biometric-based security strategy, iProov chief product and innovation officer Joe Palmer said.
The pandemic dragged companies that rely on identity verification into the future. Palmer said customers had no choice but to complete verifications remotely from their own devices on untrusted networks.
As criminals evolved, fraud strategies had to follow to keep up in the cat-and-mouse security game. Liveness detection grew out of the need to catch strategies as simple as holding a photo to a screen.
“The key to being able to verify someone remotely is not how good your face matcher is, which is an important question, but is now ultimately a solved problem when it comes to these kinds of scenarios,” Palmer explained. “It’s how well you can tell if someone is a real person or if they are copies of the victim.”
Liveness detection and digital injection attacks
Palmer sees the biggest fraud surge coming from digital injection attacks. This happens when a virtual camera or software injects digital imagery into a video stream to perform a face swap or deep fake.
The strategy’s success leans on the fact that it is hard for humans to detect webcam takeovers. Studies show roughly one in four can detect a deep fake.
Added to the fun are technologies that map faces over template overlays that digitally drape over someone’s face. The scammer can talk, blink, move and smile, all the while looking like the victim. Liveness detection systems that rely on movement are susceptible to these attacks.
Fraudsters used remote working systems and video calls to refine their approach during the pandemic. Someone with a powerful enough computer, even a decent MacBook, can complete a reasonably good face swap in real-time.
“You think you can believe your eyes, but we’re at the point where you actually can’t,” Palmer said.
How to stop fraudsters
Clues include the name of the camera being used. Does it match the user’s camera? Scammers can easily adjust, so the next step is to analyze metadata. Digital cameras act differently than virtual ones, and hints are left.
“Ultimately, it is security by obscurity because you can only do what you have access to,” Palmer explained. “A web browser is a very limited environment; it’s easier to do on iOS on Android or by installing a program on a computer because you have full access to the operating system and the camera hardware.”
But the back-and-forth continues. By running code, one can view its source and track the criminal, but they can respond by seeing what information is being collected and developing workarounds. While useful, it cannot be relied on as a security mechanism because the critical information runs on the criminal’s computer. In time, they will reverse-engineer things and find another way.
“Plenty of people will give up before trying too hard, but the serious player, organized crime, the state actors whose job it is to bypass the system will keep going until they’ve worked it all out,” Palmer said.
Another method is using AI to fight AI. Imagery can be analyzed to see if it’s been synthetically generated. iProov illuminates the face with the screen. That changes the skin, so when algorithms generate a face that is digitally draped over the criminal’s, the algorithms do not process that illumination. Palmer said iProov has successfully assessed how light does and doesn’t change once a face is illuminated.
Upgrade or fall behind
Techniques that rely on movement or speech are losing effectiveness because fraudulent algorithms constantly improve. Image-based systems that don’t have base images for reference struggle with accuracy, especially as systems improve.
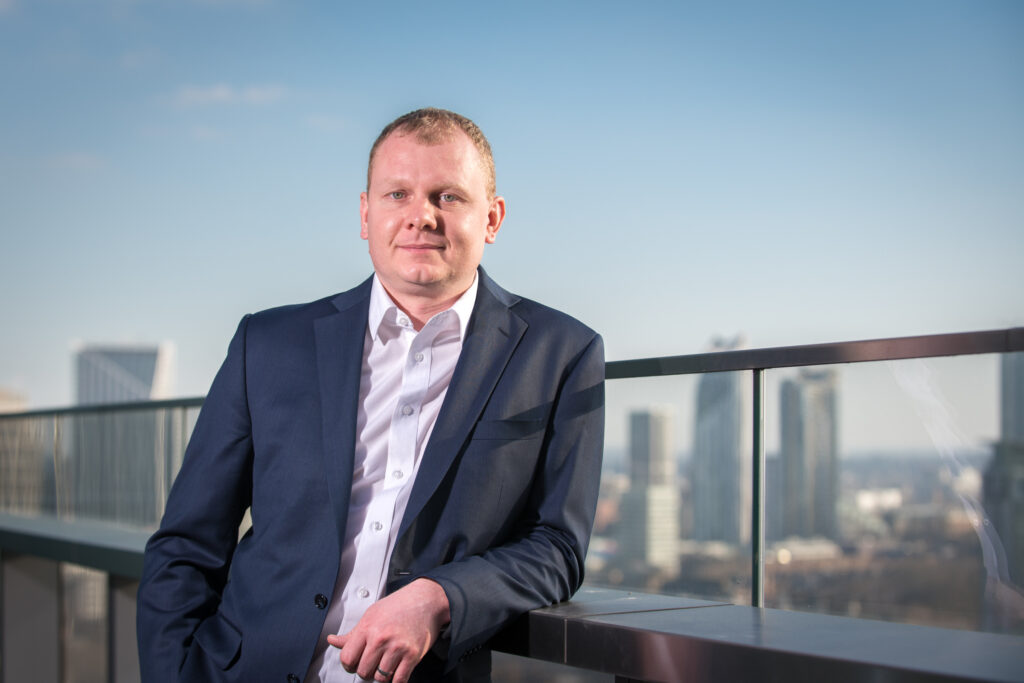
“This is very interesting work, pushing the boundaries of mathematics, encryption, and biometrics,” Palmer said. “One of the interesting things is that the world of biometrics is a probabilistic system. There is no 100% yes or no; it is a confidence score. When you match two templates together, you get a similarity score – how close are these two identities to each other? You set a threshold.”
That has its place, but Palmer stressed it doesn’t impact liveness detection. You can use the best system going, but if all a criminal has to do is hold a photo close to the camera, that system is useless.
The future focus must be on ensuring that an inbound face needing matching is a genuine version of a real person and not a deep fake.
“If you don’t detect that, then all of the clever math in the world isn’t gonna stop the attacker from getting into the system that this solution is trying to protect,” Palmer noted.
Individual risk assessment, reusable digital identities, 2024 trends to watch
In 2024, Palmer is watching for systems to understand better the risks associated with each transaction. Once determined, the system can dynamically implement the appropriate authorization level.
“The understanding of risk, the spectrum of liveness and identity assessment, and the ability to choose the right level of assessment on a per-transaction basis is going to become valuable and differentiating in the market,” Palmer said.
Look for progress in removing the rigamarole customers face when verifying their identities. They feel verification fatigue from repeatedly doing the same thing. Look for progress on reusable digital identities.
“It should be possible, and the technology now exists with verifying credentials to have a digital ID created through a robust identity proofing process and produced in a tamper-proof way,” Palmer concluded.
Also read: